The NHS AI iceberg: below the surface
- 20 May 2021
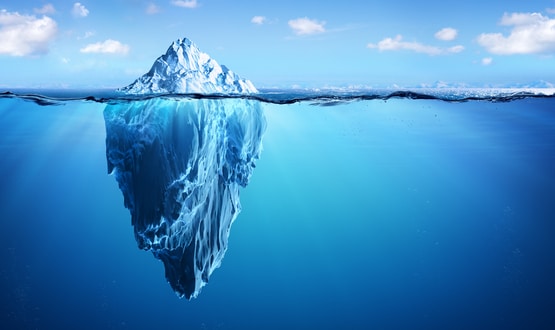
A new education focus around artificial intelligence for healthcare professionals and patients could be the way forward when it comes to the future of health. Jane Rendall from Sectra and Rachel Dunscombe, CEO of the NHS Digital Academy, explore.
A crisis point could be on the horizon for NHS imaging disciplines. Rising demand and pervasive recruitment challenges mean there will be too few experts to go around based on current ways of working.
We certainly don’t want to reach that point, and to achieve that the health service will need to adopt artificial intelligence in new ways as an important mechanism in redesigning services.
For this to happen radiologists, pathologists and other ‘ologists’ must master how AI works and how it could be used to achieve maximum impact.
These professionals, together with organisational and process experts, need to be given the headspace to work out how their profession will evolve in coming years, having taken the potential of this technology into account. They need to understand what part of their profession requires or can be strengthened by human judgement and engagement. And they need to be able to establish when decisions could be made quickly and automatically by AI.
What can be safely automated, should be automated, or have the option of being automated. More than an efficiency drive, this is a necessity to be able to deliver healthcare expected by citizens, and to facilitate early engagement and prevention.
The iceberg
There is a big education piece that needs to be undertaken in order for this complex redesign to happen effectively, and for AI to be used in more sophisticated ways than narrower diagnostic support uses often seen today.
Clinical professions are changing and will become more data driven. This will require a new skillset currently absent from learning, like understanding the technology and mathematical concepts behind algorithms.
There are four key areas where people need education and orientation, and the technology is just the tip of the iceberg.
- Around the tech – the tip of the iceberg. Clinicians need to have an understanding of how to read and interpret results from AI applications, and a vision of what exists, what AI can do, what is emerging and what it could do in the future. There is then a huge amount underneath the surface. Principally, the remaining three areas.
- Governance and quality assurance – how quality works in an AI environment and the development of continuous quality assurance in institutions. It is important to understand how an algorithm performs on a certain patient population within an institution and how that evolves over time. Being in control and understanding how algorithms behave will be key for institutions.
- Workflow redesign – changing how clinicians work and augment themselves as professionals.
- At the system and patient level – pathway redesign to leverage all of the above. This might be around patients going to an MRI scanner that is nearer. Or they might get an automatic text message with results, or access to preliminary findings before they leave hospital. Pathway redesign is essential to un-constrain healthcare for the patient.
Unless we tackle this iceberg whole, we won’t achieve impact at scale and pace – instead we risk creating orphaned silos of technology that don’t fit into the healthcare system.
That’s why this needs to be part of continuous professional development and education for anyone in healthcare using AI. People need to understand what problems they are trying to solve, and ways in which that can be done safely.
Educating patients
When talking pathway redesign our radiologists, pathologists and others will need to understand how this AI is communicated to citizens. That includes the explanations that patients see, the outcomes and measures patients see, and informing choices presented to the patient, potentially via their patient portal. Many patients already get choices around how they receive information; this could extend to their diagnostic choices.
A potential future option to have a preliminary diagnosis in 30 seconds by choosing to use an algorithm to look at your image, rather than 15 days for a human counterpart to examine it, could be a valid option in many cases.
And if we can gather evidence over time of the efficacy of those choices, we can show that to patients.
We can move from prescribing a set of pathways to citizens to giving them more choice, to informing how they interact with an algorithm.
Conversely some patients might have a complex history and prefer an analogue approach. Patients might be advised to rely on a radiologist for complex cases. But for a relatively simple bone break, you might choose an algorithm. Humans add most value where there is complexity. Some of this is about choice, some will be about advice. And part of this equation is about determining where choice is appropriate.
Digitally ready workforce
This is transformation – it is about how we are going to practice medicine or radiology in the future – not orphaning tech along the way.
It is about empowering a digital and AI ready workforce to reimagine their own careers, their workplace and workflow.
The potential crisis point creates a sense of urgency, but this is also an opportunity to make service redesign everyone’s job – so they are not just part of the service, they are part of the future.
3 Comments
The buzzwords (headspace, engagement, citizens, ‘around’) in this article are irritating.
The subtitle “Educating Patients” added to my ire.
I persisted.
Then I got to the paragraph suggesting that ‘…patients might … prefer an analogue approach.’).
Oh dear.
This article may be of interest.
https://www.forbes.com/sites/saibala/2020/02/03/artificial-intelligence-is-not-ready-for-the-intricacies-of-radiology/
Who is liable for AI errors? patients like someone to blame and/or sue.
In imaging, it would need to replace radiologists for a significant number of cases. This may initially be for certain modalities or investigations. CXR for ? lung cancer has been cited recently. But as well as identifying the presence or not of the big C, other abnormalities might be present, that will need to appear in the report. can the cancer-AI robot do that? Who gets the blame if something non-ca but clinically relevant is missed? the hospital that bought the AI, the company that built it and/or trained it?
There is no point in getting the AI reports checked by a proper radiologist, as that takes as much time as just reporting it, so no solution to the manpower crisis.
I imagine that if the AI says ‘i don’t know what this is’ it would pass the exam to the radiologists’ lists, so it reduces workload by the number of exams it feels able to report – but will that make a significant difference?
Will these reports need re-reporting before the MDT so the radiologist can say something useful about it? Not much workload reduction in that use case, then.
It sounds like a good concept, but would need to be able to REPLACE a radiologist, rather than just giving a first impression that needs confirmation.
Comments are closed.