Advisory Series: Artificial Intelligence
- 19 September 2019
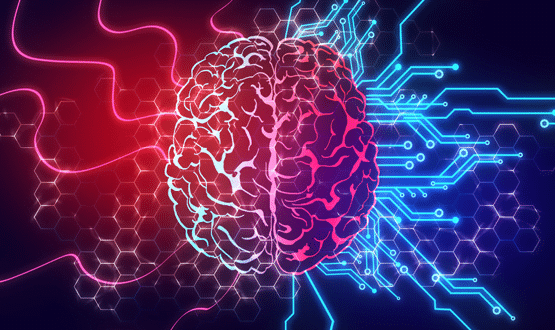
Artificial Intelligence – Advisory Series, September 2019
Contents focus
Get a targeted view by selecting your chosen topic from the Artificial Intelligence Advisory list below:
By Kim Thomas – Digital Health
Artificial intelligence (AI) has long been promoted as a tool which could transform the way clinicians work in the NHS. However there is an awareness that the space between the promise and the reality is often large. Kim Thomas reports on the realities and myths surrounding AI in healthcare.
Great Ormond Street Hospital (GOSH), in common with other NHS hospitals, has a problem with patients not attending appointments.
As Neil Sebire, chief research officer and director of the hospital’s Digital Research, Informatics and Virtual Environment (DRIVE) unit points out: “If you book a clinic and 20% of the patients don’t turn up, you’re wasting 20% of the slots.”
Could artificial intelligence (AI) – which uses software to perform tasks usually requiring human intelligence – make a difference?
Getting the most out of algorithms
By analysing operational data, GOSH hopes to develop an algorithm that can predict which patients are likely to miss an appointment, enabling the hospital to find ways to maximise the likelihood of them attending.
Another AI project, yet to start, involves analysing anonymised patient data to predict the effect of a particular drug on certain groups of patients according to variables such as age or sex.
“Instead of saying 5% of patients who take this drug will be nauseous, can we model that there’s a 45% chance that this patient will be nauseous, whereas for this patient it’s a very low chance,” Sebire explains.
Once the algorithm has been built, it can be refined.
Sebire points out: “As long as you’ve got data, the model can get more and more complicated and take account of more and more factors.”
Looking at the national picture
In August, health secretary Matt Hancock announced an investment of £250m to create a National AI Lab for the NHS.
The announcement followed publication of the government’s Topol Review in February, which said that AI “has the potential to transform the delivery of healthcare in the NHS, from streamlining workflow processes to improving the accuracy of diagnosis and personalising treatment, as well as helping staff work more efficiently and effectively”.
In practice, AI projects already underway in the NHS tend to be small-scale and focused, like the GOSH projects, on improving operational efficiencies.
Flagging up the important bits
Bradford Teaching Hospitals NHS Foundation Trust, for example, has been working with the University of Bradford to develop algorithms based on Secondary Uses Service (SUS) data to predict how long patients will stay, and to identify early those patients who may end up staying longer than necessary (because a social care plan has to be put in place, for example).
Another project will look at how to identify A&E patients most likely to require a test, enabling it to be ordered immediately rather than after a long wait by the patient.
The hospital also intends to use data from its electronic patient record (EPR) to develop algorithms that can guide clinicians – but Tom Lawton, head of clinical artificial intelligence, believes that AI is a long way from being able to replace clinical decision-making.
It can, however, alert doctors to significant symptoms.
“If an AI system can just help you pick out the important bits and flag things up to you, that makes the situation safer because you’re not missing stuff,” Lawton says.
Not as simple as you think
Nonetheless, some projects are being undertaken to improve clinical outcomes. The Royal Free NHS Foundation Trust has successfully been using Google DeepMind Health’s AI app Streams to predict which patients might be at risk of acute kidney injury – though it has been criticised for sharing patient data with the company.
Moorfields Eye Hospital NHS Foundation Trust also has a partnership with DeepMind Health to use AI to analyse eye scans for signs of disease.
The technology, which has been based on the analysis of thousands of anonymised eye scans, has shown an effectiveness rate of 94%, matching human experts.
Currently waiting times to see an ophthalmologist are long, and the aim is to use the technology to prioritise patients who need to be treated urgently.
Despite the promising results, the technology is still a few years away from being used in practice, says Dawn Sim, director of telemedicine at Moorfields.
“The next steps are to validate the algorithm in a multi-centre study and that is under way,” she says.
“And then the step up from that would be implementing it and then scaling it, looking at how we’re doing and refining the algorithm.
“It’s not quite as simple as: ‘We have a finished product that is ready to roll.'”
Unexpected benefits
The use of AI to speed up diagnosis of radiology scans has long been seen as one of the most promising clinical applications.
At East Kent Hospitals University NHS Foundation Trust, Neelan Das, consultant cardiac and interventional radiologist and lead for AI at the trust, is leading a team piloting software from Qure.Ai that automates the reading of chest X-rays, identifying the ones that need urgent treatment.
The algorithm used by the software has been trained on 1.2 million X-rays, and it has been used successfully in India to screen for tuberculosis, Das says.
In the East Kent pilot, the technology will act as a “workflow prioritisation tool” though a radiologist would still need to check the results.
Currently, Das points out, the shortage of radiologists means that chest X-rays are often read by the doctor ordering the X-ray – not just in East Kent, but elsewhere in the NHS.
So if the trust decides to implement the Qure.AI software, rather than saving time, it will create work for radiologists. The potential benefit comes not from reducing workload, but from improving the quality of diagnosis.
Das has concerns about the implementation of AI, however. A CT chest scan, for example, has multiple components, including lungs, ribs, blood vessels, muscles and trachea – but an AI application will typically look only at one aspect, such as lung nodules.
Backing up the evidence
While that has the potential to be extremely useful, it’s not enough on its own.
“For one section of the body you might need 10 or 12 different AI providers – it’s impractical to implement them,” Das says.
He worries, too, about quality, arguing all AI apps should undergo trials and the results published in peer-reviewed journals.
AI apps are continually evolving through usage, with Das adding: “If an AI app passes accreditation once, should it undergo a process of accreditation again a year later?”
The government’s own code of conduct, he notes, identifies important principles for implementing AI safely.
Unresolved questions
There are other barriers to overcome before widespread adoption of AI in the NHS. Some questions are still unresolved.
Hugh Whittall, director of the Nuffield Council on Bioethics, points to the questions of trust and privacy surrounding the use of health data on a large scale, but there is an added difficulty, he notes, in creating algorithms based on data sources that may not be representative of the wider population.
Another difficulty comes, says Sebire, “when you have a complicated AI system that takes a huge amount of data and then comes up with a recommendation, and we as humans cannot understand how it came up with that recommendation. The question in those scenarios is: do you just go with the machine, or do you go with the human?”
Whittall agrees, adding: “It does raise questions about the responsibility of the doctor, where legal liability might lie, whether you are simply undermining the professional status of the health professionals and whether patients’ decision making and patient consent can ever be properly informed if the decision or recommendation can’t be explained adequately.”
Looking beyond the hype
Despite the hype, we are a long way from a world in which apps, rather than doctors, are making clinical decisions.
They do, however, have the potential to bring efficiencies and to speed up decision-making.
As Sebire says: “There is so much benefit for the NHS from simply improving operational efficiencies that you don’t need to go down the route of robot doctors diagnosing patients to get a massive amount of benefit.”
How to address the “data-wrangling issue”
By InterSystems
AI has the potential to transform the way healthcare is delivered in the NHS. By applying algorithms to large data sets, it is possible to make diagnoses or predict the likelihood of individuals developing particular diseases.
The implementation of AI, however, requires substantial amounts of data. In the NHS, data is usually held in multiple clinical and administrative databases, often in different formats, making it hard to access and manage.
Data may be incomplete or inaccurate, so that creating a single, coherent, usable data set is time-consuming. Even a task such as deciding whether a care record from two different clinical systems refers to the same patient can be laborious. About 80% of data scientists’ time is spent on “data wrangling” – the job of converting raw data into something useful for AI purposes.
Making data usable
InterSystems addresses this through IRIS, a data platform that simplifies the job of taking data from multiple sources and making it usable.
HealthShare, the regional care record solution from InterSystems, now uses IRIS to amalgamate patient healthcare information from different sources.
It is then relatively straightforward for customers to apply AI algorithms to the data.
Jon Payne, manager for sales engineering at InterSystems, says one US customer is using population-level data to look at the risk of patients developing diabetes, for example, or of going to the emergency department. The customer is then able to “develop appropriate care plans or look at ways to improve patients’ health”.
Another InterSystems customer, says Payne, is a pathology lab combining genetic data with clinical data to improve predictions about whether a genetic mutation is likely to result in an individual developing cancer.
Other customers are using AI to analyse data from hundreds of thousands of smart home drug delivery devices.
“These provide information, not only about whether someone has taken a drug, but how effectively they’re using the device, such as flow rates for inhalers,” says Payne. “So you can look at the efficiency and effectiveness of the devices on a wider scale.”
Getting data protection right
Data protection laws mean that widescale adoption of AI by the NHS may take some time, says Payne. There is plenty of potential, however, to use it for operational purposes, such as predicting the availability of lab equipment.
“Introducing change in that context can make a big difference and could be a quick win for the NHS,” he says.
![]() |
|
Contact number: 017 5382 9647 Email: Erica.Bennett@InterSystems.com Our website: www.intersystems.com/uk/ Twitter: @InterSystemsUK LinkedIn: www.linkedin.com/company/intersystems-uk/ Facebook: www.facebook.com/InterSystems/ [ssba]
|
Addressing the slow uptake of AI
By Sectra
At a time when the NHS is short of radiologists, AI has the ability to make the process of interpreting radiology images much more efficient and also decrease the rate of errors.
If AI algorithms are trained and designed correctly, they could significantly increase productivity for radiologist, says Chris Scarisbrick, sales director at Sectra. So what is holding back the adoption of AI?
There are two major reasons for the slow take-up. One is the lack of tight integration into the existing workflow. Radiologists are already dealing with a picture archiving and communications system (PACS), and many early AI apps require manual interactions that add to the workload of clinicians who are already overburdened.
The second is that the traditional model for procuring healthcare IT in the NHS doesn’t work well for AI. Procurement is a time-intensive process that usually results in a supplier being chosen to provide services for a minimum of five years. But many AI suppliers are start-ups, and more are entering the market all the time. This means NHS trusts require a more flexible approach so that they can take advantage of the latest technologies or acquire such apps from a platform provider.
Getting happy with apps
Sectra has addressed both those problems by doing much of the difficult work upfront. It is piloting an AI app store, with the company quality assuring the integration with AI apps to make sure they are tested and are efficient in the workflow. All the apps are integrated seamlessly into the Sectra PACS, meaning the workflow of radiologists is not disrupted. They also integrate with apps from many different vendors.
Scarisbrick gives an example of what kinds of apps are available in a pilot version of the store.
There are apps which are able to evaluate a range of examinations types for a wide range of conditions. In this way, a whole worklist of CT scans, chest X-rays or brain scans (for example) can be assessed by the AI, and it can feed these findings back to the Sectra PACS. The Sectra PACS has the logic within to sort the worklist based upon the clinical severity so that the clinician is served up with the most urgent cases first.
Another app on the market will be able to perform the second read of double-read studies, potentially offering significant time savings without compromising on quality.
Sectra is now working towards a pilot of an AI app store with selected NHS customers. This will overcome many of the problems associated with the procurement of the technology and will help to drive the adoption of AI in radiology, but also in other imaging heavy specialties such as pathology.
“AI does have the potential to be transformative and drive efficiencies, we just need to make it easier for physicians to adopt and benefit from these tools, and feel comfortable by the results they provide” says Scarisbrick.
![]() |
|
Contact number: 012 7921 3653 Email: caroline.marjason@sectra.com Our website: www.sectra.com/medical Twitter: @SectraNews LinkedIn: www.linkedin.com/company/sectra [ssba]
|